Downscaled High Resolution Datasets for Climate Change Projections
Global climate models (GCMs) have generated projections of temperature, precipitation and other important climate change parameters with spatial resolutions of 100 to 300 km. However, higher spatial resolution information is required to assess threats to individual installations or regions. A variety of “downscaling” approaches have been used to produce high spatial resolution output (datasets) from the global climate models at scales that are useful for evaluating potential threats to critical infrastructure at regional and local scales. These datasets enable development of information about projections produced from various climate models, about downscaling to achieve desired locational specificity, and about selecting the appropriate dataset(s) to use for performing specific assessments. This article describes how these datasets can be accessed and used to evaluate potential climate change impacts.
Related Article(s):
Contributor(s): Dr. Rao Kotamarthi
Key Resource(s):
- Use of Climate Information for Decision-Making and Impacts Research: State of our Understanding[1]
- Applying Climate Change Information to Hydrologic and Coastal Design of Transportation Infrastructure, Design Practices[2]
- Statistical Downscaling and Bias Correction for Climate Research[3]
- Downscaling Techniques for High-Resolution Climate Projections: From Global Change to Local Impacts[4]
Downscaling of Global Climate Models
Some communities and businesses have begun to improve their resilience to climate change by building adaptation plans based on national scale climate datatsets (National Adaptation Plans), regional datasets (New York State Flood Risk Management Guidance[5]), and datasets generated at local spatial resolutions. Resilience to the changing climate has also been identified by the US Department of Defense (DoD) as a necessary part of the installation planning and basing process (DoD Report on Effects of a Changing Climate[6]). More than 79 installations were identified as facing potential threats from climate change. The threats faced due to changing climate include recurrent flooding, droughts, desertification, wildfires and thawing permafrost.
Assessing the threats climate change poses at regional and local scales requires data with higher spatial resolution than is currently available from global climate models. Global-scale climate models typically have spatial resolutions of 100 to 300 km, and output from these models needs to be spatially and/or temporally disaggregated in order to be useful in performing assessments at smaller scales. The process of producing higher spatial-temporal resolution climate model output from coarser global climate model outputs is referred to as “downscaling” and results in climate change projections (datasets) at scales that are useful for evaluating potential threats to regional and local communities and businesses. These datasets provide information on temperature, precipitation and a variety of other climate variables for current and future climate conditions under various greenhouse gas (GHG) emission scenarios. There are a variety of web-based tools available for accessing these datasets to evaluate potential climate change impacts at regional and local scales.
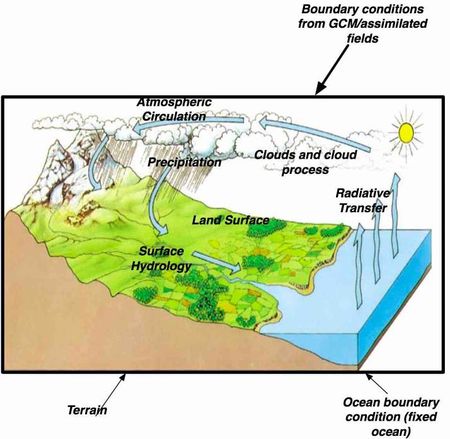
Methods for Downscaling
Dynamical Downscaling | Statistical Downscaling |
---|---|
Deterministic climate change simulations that output many climate variables with sub-daily information |
Primarily limited to daily temperature and precipitation |
Computationally expensive; hence, limited number of simulations – both GHG emission scenarios and global climate models downscaled |
Computationally efficient; hence, downscaled data typically available for many different global climate models and GHG emission scenarios |
May require additional bias correction | Method incorporates bias correction |
Observational data at the downscaled location are not necessary to obtain the downscaled output at the location |
Best suited for locations with 30 years or more of observational data |
Does not assume stationarity or in other words the model simulates the future regardless of what has happened in the past |
Stationarity assumption - assumes that the statistical relationship between global climate model and observations will remain constant in the future |
There are two main approaches to downscaling. One method, commonly referred to as “statistical downscaling”, uses the empirical-statistical relationships between large-scale weather phenomena and historical local weather data. In this method, these statistical relationships are applied to output generated by global climate models. A second method uses physics-based numerical models (regional-scale climate models or RCMs) of weather and climate that operate over a limited region of the earth (e.g., North America) and at spatial resolutions that are typically 3 to 10 times finer than the global-scale climate models. This method is known as “dynamical downscaling”. These regional-scale climate models are similar to the global models with respect to their reliance on the principles of physics, but because they operate over only part of the earth, they require information about what is coming in from the rest of the earth as well as what is going out of the limited region of the model. This is generally obtained from a global model. The primary differences between statistical and dynamical downscaling methods are summarized in Table 1.
It is important to realize that there is no “best” downscaling method or dataset, and that the best method/dataset for a given problem depends on that problem’s specific needs. Several data products based on downscaling higher level spatial data are available (USGS, MACA, NARCCAP, CORDEX-NA). The appropriate method and dataset to use depends on the intended application. The method selected should be able to credibly resolve spatial and temporal scales relevant for the application. For example, to develop a risk analysis of frequent flooding, the data product chosen should include precipitation at greater than a diurnal frequency and over multi-decadal timescales. This kind of product is most likely to be available using the dynamical downscaling method. SERDP reviewed the various advantages and disadvantages of using each type of downscaling method and downscaling dataset, and developed a recommended process that is publicly available[1]. In general, the following recommendations should be considered in order to pick the right downscaled dataset for a given analysis:
- When a problem depends on using a large number of climate models and emission scenarios to perform preliminary assessments and to understand the uncertainty range of projections, then using a statistical downscaled dataset is recommended.
- When the assessment needs a more extensive parameter list or is analyzing a region with few long-term observational data, dynamically downscaled climate change projections are recommended.
Uncertainty in Projections
Model or Dataset Name |
Model Method |
Output Variables |
Output Format |
Spatial Resolution |
Time Resolution |
---|---|---|---|---|---|
Statistical Downscaled Datasets | |||||
WorldClim[7] | Delta | T(min, max, avg), Pr |
NetCDF | grid: 30 arc sec to 10 arc min |
month |
Bias Corrected / Spatial Disaggregation (BCSD)[8] |
Empirical Quantile Mapping |
Runoff, Streamflow |
NetCDF | grid: 7.5 arc min | day |
Asynchronous Regional Regression Model (ARRM v.1)[9] |
Parameterized Quantile Mapping |
T(min, max), Pr | NetCDF | stations plus grid: 7.5 arc min |
day |
Statistical Downscaling Model (SDSM)[10] | Weather Generator | T(min, max), Pr | PC Code | stations | day |
Multivariate Adaptive Constructed Analogs (MACA)[11] |
Constructed Analogues | 10 Variables | NetCDF | grid: 2.5 arc min | day |
Localized Constructed Analogs (LOCA)[12] | Constructed Analogues | T(min, max), Pr | NetCDF | grid: 3.75 arc min | day |
NASA Earth Exchange Downscaled Climate Projections (NEX-DCP30)[8] |
Bias Correction / Spatial Disaggregation |
T(min, max), Pr | NetCDF | grid: 30 arc sec | month |
Dynamical Downscaled Datasets | |||||
North American Regional Climate Change Assessment Program (NARCCAP)[13] |
Multiple Models | 49 Variables | NetCDF | grid: 30 arc min | 3 hours |
Coordinated Regional Climate Downscaling Experiment (CORDEX)[14] |
Multiple Models | 66 Variables | NetCDF | grid: 30 arc min | 3 hours |
Strategic Environmental Research and Development Program (SERDP)[15] |
Weather Research and Forecasting (WRF v3.3) |
80+ Variables | NetCDF | grid: 6.5 arc min | 3 hours |
A primary cause of uncertainty in climate change projections, especially beyond 30 years into the future, is the uncertainty in the greenhouse gas (GHG) emission scenarios used to make climate model projections. The best method of accounting for this type of uncertainty is to apply a climate change model to multiple GHG emission scenarios (see also: Wikipedia: Representative Concentration Pathway).
The uncertainties in climate projections over shorter timescales, less than 30 years out, are dominated by something known as “internal variability” in the models. Different approaches are used to address the uncertainty from internal variability[4]. A third type of uncertainty in climate modeling, known as scientific uncertainty, comes from our inability to numerically solve every aspect of the complex earth system. We expect this scientific uncertainty to decrease as we understand more of the earth system and improve its representation in our numerical models. As discussed in Climate Change Primer, numerical experiments based on global climate models are designed to address these uncertainties in various ways. Downscaling methods evaluate this uncertainty by using several independent regional climate models to generate future projections, with the expectation that each of these models will capture some aspects of the physics better than the others, and that by using several different models, we can estimate the range of this uncertainty. Thus, the commonly accepted methods for accounting for uncertainty in climate model projections are either using projections from one model for several emission scenarios, or applying multiple models to project a single scenario.
A comparison of the currently available methods and their characteristics is provided in Table 2 (adapted from Kotamarthi et al., 2016[1]). The table lists the various methodologies and models used for producing downscaled data, and the climate variables that these methods produce. These datasets are mostly available for download from the data servers and websites listed in the table and in a few cases by contacting the respective source organizations.
The most popular and widely used format for atmospheric and climate science is known as NetCDF, which stands for Network Common Data Form. NetCDF is a self-describing data format that saves data in a binary format. The format is self-describing in that a metadata listing is part of every file that describes all the data attributes, such as dimensions, units and data size and in principal should not need additional information to extract the required data for analysis with the right software. However, specially built software for reading and extracting data from these binary files is necessary for making visualizations and further analysis. Software packages for reading and writing NetCDF datasets and for generating visualizations from these datasets are widely available and obtained free of cost (NetCDF-tools). Popular geospatial analysis tools such as ARC-GIS, statistical packages such as ‘R’ and programming languages such as Fortran, C++, and Python have built in libraries that can be used to directly read NetCDF files for visualization and analysis.
References
- ^ 1.0 1.1 1.2 1.3 1.4 Kotamarthi, R., Mearns, L., Hayhoe, K., Castro, C.L., and Wuebble, D., 2016. Use of Climate Information for Decision-Making and Impacts Research: State of Our Understanding. Department of Defense, Strategic Environmental Research and Development Program (SERDP), 55pp. Free download from: SERDP-ESTCP
- ^ Kilgore, R., Thomas, W.O. Jr., Douglass, S., Webb, B., Hayhoe, K., Stoner, A., Jacobs, J.M., Thompson, D.B., Herrmann, G.R., Douglas, E., and Anderson, C., 2019. Applying Climate Change Information to Hydrologic and Coastal Design of Transportation Infrastructure, Design Practices. The National Cooperative Highway Research Program, Transportation Research Board, Project 15-61, 154 pages. Free download from: The Transportation Research Board
- ^ Maraun, D., and Wildmann, M., 2018. Statistical Downscaling and Bias Correction for Climate Research. Cambridge University Press, Cambridge, UK. 347 pages. DOI: 10.1017/9781107588783 ISBN: 978-1-107-06605-2
- ^ 4.0 4.1 Kotamarthi, R., Hayhoe, K., Wuebbles, D., Mearns, L.O., Jacobs, J. and Jurado, J., 2021. Downscaling Techniques for High-Resolution Climate Projections: From Global Change to Local Impacts. Cambridge University Press, Cambridge, UK. 202 pages. DOI: 10.1017/9781108601269 ISBN: 978-1-108-47375-0
- ^ New York State Department of Environmental Conservation, 2020. New York State Flood Risk Management Guidance for Implementation of the Community Risk and Resiliency Act. Free download from: New York State Report.pdf
- ^ US Department of Defense, 2019. Report on Effects of a Changing Climate to the Department of Defense. Free download from: DoD Report.pdf
- ^ Hijmans, R.J., Cameron, S.E., Parra, J.L., Jones, P.G. and Jarvis, A., 2005. Very High Resolution Interpolated Climate Surfaces for Global Land Areas. International Journal of Climatology: A Journal of the Royal Meteorological Society, 25(15), pp 1965-1978. DOI: 10.1002/joc.1276
- ^ 8.0 8.1 Wood, A.W., Maurer, E.P., Kumar, A. and Lettenmaier, D.P., 2002. Long‐range experimental hydrologic forecasting for the eastern United States. Journal of Geophysical Research: Atmospheres, 107(D20), 4429, pp. ACL6 1-15. DOI:10.1029/2001JD000659 Free access article available from: American Geophysical Union Report.pdf
- ^ Stoner, A.M., Hayhoe, K., Yang, X., and Wuebbles, D.J., 2013. An Asynchronous Regional Regression Model for Statistical Downscaling of Daily Climate Variables. International Journal of Climatology, 33(11), pp. 2473-2494. DOI:10.1002/joc.3603
- ^ Wilby, R.L., and Dawson, C.W., 2013. The Statistical DownScaling Model: insights from one decade of application. International Journal of Climatology, 33(7), pp. 1707-1719. DOI: 10.1002/joc.3544
- ^ Hidalgo, H.G., Dettinger, M.D. and Cayan, D.R., 2008. Downscaling with Constructed Analogues: Daily Precipitation and Temperature Fields Over the United States. California Energy Commission PIER Final Project, Report CEC-500-2007-123. Report.pdf
- ^ Pierce, D.W., Cayan, D.R. and Thrasher, B.L., 2014. Statistical Downscaling Using Localized Constructed Analogs (LOCA). Journal of Hydrometeorology, 15(6), pp. 2558-2585. DOI: 10.1175/JHM-D-14-0082.1 Free access article available from: American Meteorological Society. Report.pdf
- ^ Mearns, L.O., Gutowski, W., Jones, R., Leung, R., McGinnis, S., Nunes, A. and Qian, Y., 2009. A Regional Climate Change Assessment Program for North America. Eos, Transactions, American Geophysical Union, 90(36), p.311. DOI: 10.1029/2009EO360002 Free access article from: American Geophysical Union Report.pdf
- ^ Giorgi, F., Jones, C., and Asrar, G.R., 2009. Addressing climate information needs at the regional level: the CORDEX framework. World Meteorological Organization (WMO) Bulletin, 58(3), pp. 175-183. Free access article from: World Meteorological Organization Report.pdf
- ^ Wang, J., and Kotamarthi, V.R., 2015. High‐resolution dynamically downscaled projections of precipitation in the mid and late 21st century over North America. Earth's Future, 3(7), pp. 268-288. DOI: 10.1002/2015EF000304 Free access article from: American Geophysical Union Report.pdf
See Also
Climate Change Impacts to Department of Defense Installations, SERDP Project RC-2242